Meta Exec Shuts Down Shocking Llama 4 Benchmark Score Manipulation Rumors
0
0
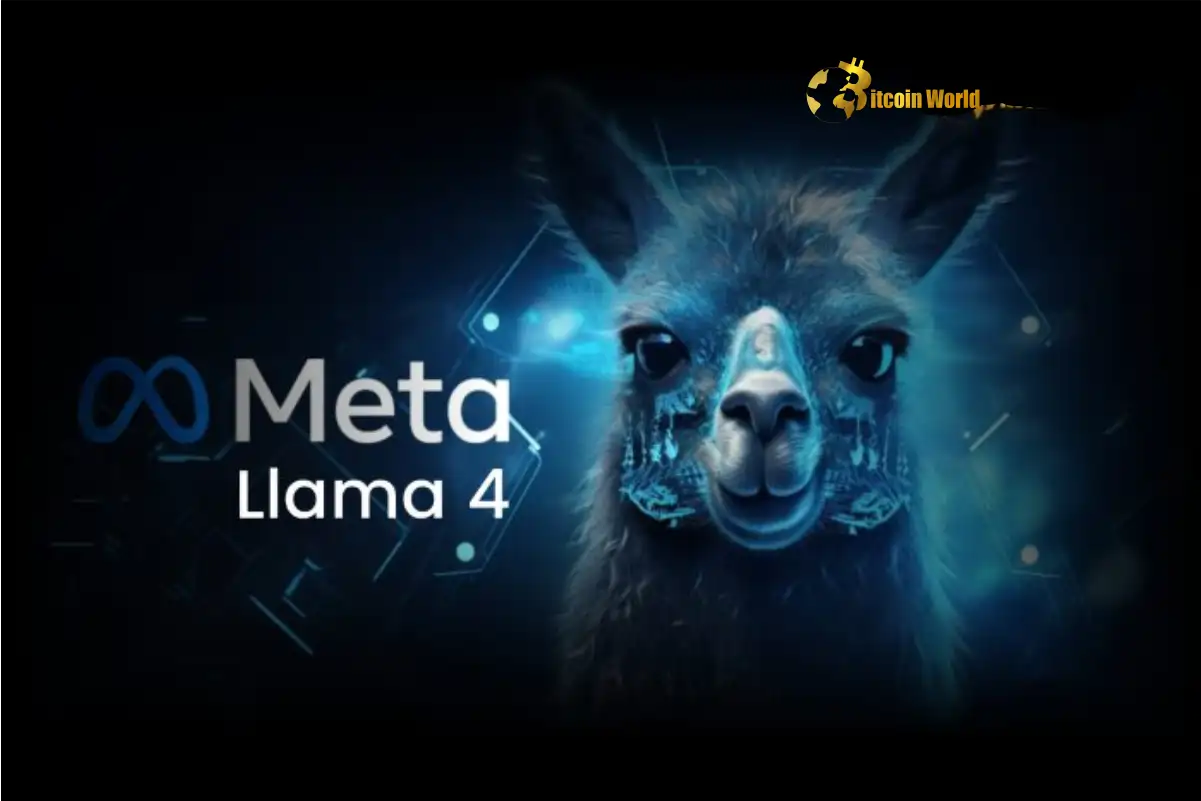
Is Meta playing fair in the AI arena? Whispers of deceit have emerged, casting a shadow over the much-hyped Llama 4 AI model. Crypto and tech enthusiasts alike are watching closely as accusations fly, questioning the integrity of Meta’s latest AI marvel. Did they truly boost AI benchmarks artificially, or is this just another case of online speculation gone wild? Let’s dive into the heart of this controversy and uncover the facts.
Did Meta Deceptively Inflate Llama 4’s AI Benchmarks?
Over the weekend, the internet buzzed with rumors suggesting that Meta, the tech giant behind Facebook and Instagram, had strategically trained its new AI models, specifically Llama 4 Maverick and Llama 4 Scout, to excel in AI benchmarks. The accusation? That this training was designed to mask potential weaknesses and present a misleadingly positive picture of the models’ capabilities. This explosive claim, originating from a disgruntled ex-Meta employee on a Chinese social media platform, quickly spread across X and Reddit, igniting a firestorm of debate within the AI and tech communities.
The core of the accusation revolves around the concept of ‘test sets’ in AI benchmarks. Think of test sets as the final exam for an AI model. They are datasets used to evaluate how well a model performs *after* it has been trained. If a company were to train its model *on* these test sets, it would be akin to giving the model the answer key before the exam. This practice, if true, would artificially inflate the benchmark scores, making the model seem far more competent than it actually is in real-world applications.
Meta’s Swift Denial: Setting the Record Straight
In response to the escalating rumors, Ahmad Al-Dahle, Meta’s VP of generative AI, wasted no time in issuing a firm denial. Taking to X, Al-Dahle stated unequivocally that the allegations were “simply not true.” He explicitly refuted the claim that Meta trained its Llama 4 Maverick and Llama 4 Scout models on test sets used for AI benchmarks. This direct and public denial is a crucial step in damage control, but does it fully quell the rising tide of skepticism?
Fueling the Fire: Reports of Inconsistent Performance
Several factors have contributed to the rapid spread and persistence of these rumors:
- Whispers of Weaknesses: Reports have surfaced suggesting that Maverick and Scout, despite their impressive benchmark scores, exhibit less-than-stellar performance in certain practical tasks. This discrepancy between benchmark results and real-world application has understandably raised eyebrows.
- The LM Arena Factor: Meta’s decision to showcase an experimental, unreleased version of Maverick on the popular LM Arena AI benchmark platform has further fueled suspicion. Researchers observing the publicly available Maverick versus the version on LM Arena have reported noticeable differences in behavior and performance.
- Mixed Quality Across Platforms: Al-Dahle himself acknowledged user reports of “mixed quality” from Maverick and Scout when accessed through different cloud providers. While he attributed this to the initial rollout phase and ongoing optimizations, it nonetheless adds to the perception of inconsistency.
The Race to Deployment: Speed vs. Stability?
Al-Dahle’s explanation for the reported inconsistencies points to a rapid deployment strategy. He stated that Meta released the Llama 4 models “as soon as they were ready,” anticipating that it would take several days for public implementations to fully stabilize across various platforms. This “release early, iterate quickly” approach is common in the tech world, but in the sensitive domain of AI models, especially those touted as groundbreaking, it can invite scrutiny and skepticism if not managed transparently.
What Does This Mean for the Future of AI Transparency?
This episode highlights the growing importance of transparency and accountability in the development and evaluation of AI models. As AI becomes increasingly integrated into various aspects of our lives, from cryptocurrency trading algorithms to everyday applications, the need for trust and verifiable performance metrics becomes paramount.
Here are some key takeaways from this unfolding situation:
- Benchmark Skepticism: The incident underscores the potential limitations of relying solely on AI benchmarks as definitive measures of model capability. While benchmarks provide a standardized way to compare models, they can be susceptible to manipulation or may not fully reflect real-world performance.
- The Power of Community Scrutiny: The rapid spread of rumors and the ensuing public discourse demonstrate the power of online communities, particularly within the AI research and open-source spaces, to scrutinize and hold tech giants accountable.
- Need for Clear Communication: Meta’s prompt response is commendable, but going forward, proactive and transparent communication regarding model development, evaluation methodologies, and potential limitations will be crucial for building and maintaining trust.
Navigating the AI Hype Cycle: A Word of Caution
The AI landscape is rife with hype and bold claims. While advancements in AI models like Llama 4 are genuinely exciting and hold immense potential, it’s essential to approach them with a healthy dose of skepticism. Don’t be swayed by inflated benchmark scores alone. Look for independent evaluations, real-world performance data, and transparent reporting from developers. The true measure of an AI model’s success lies not just in its ability to ace a test, but in its practical utility and positive impact on the world.
The controversy surrounding Llama 4’s benchmark scores serves as a potent reminder: in the rapidly evolving world of AI, critical thinking and informed analysis are more vital than ever. As the technology matures and its influence expands, demanding transparency and rigorous evaluation will be key to ensuring responsible and beneficial AI development.
To learn more about the latest AI market trends, explore our article on key developments shaping AI features.
0
0
Securely connect the portfolio you’re using to start.