Revealing Truth: Are Meta’s AI Benchmarks for Maverick Model Misleading?
0
0
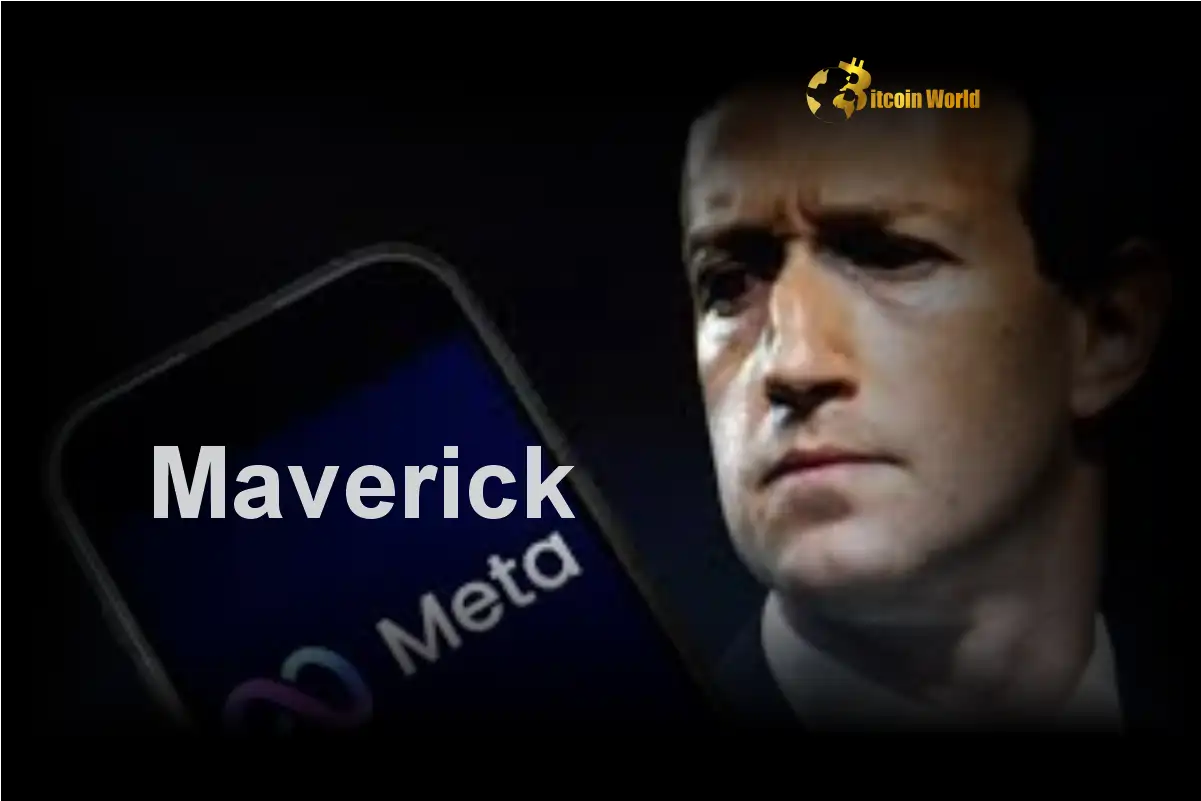
The world of Artificial Intelligence is constantly evolving, with new models and breakthroughs announced almost daily. For cryptocurrency enthusiasts and investors tracking AI’s impact on blockchain and decentralized technologies, understanding the true capabilities of these AI models is crucial. Recently, Meta, a tech giant increasingly involved in the AI space, unveiled its new flagship AI model, Maverick. Initial reports placed Maverick high in AI benchmark rankings, specifically on the LM Arena platform. But, is everything as it seems? Let’s dive into the revealing details and uncover potential discrepancies in Meta’s AI benchmarks for Maverick.
Are Meta’s Maverick AI Model Benchmarks on LM Arena Genuinely Representative?
When Meta launched Maverick, it quickly climbed to the second spot on the LM Arena leaderboard. This ranking, based on human evaluations comparing different AI model outputs, initially suggested Maverick was a top-tier performer. However, eagle-eyed AI researchers soon noticed something amiss. It appears the version of Maverick showcased on LM Arena, dubbed an “experimental chat version” by Meta itself, isn’t the same as the publicly accessible version for developers. This distinction raises serious questions about the validity of these AI benchmarks and their relevance for practical applications.
Here’s a breakdown of the key concerns:
- Customized for Benchmarks: Meta’s own Llama website states that the LM Arena testing utilized “Llama 4 Maverick optimized for conversationality.” This suggests a tailored version specifically designed to excel in LM Arena’s evaluation format.
- Benchmark Reliability Questioned: LM Arena, while popular, has faced scrutiny regarding its reliability as a definitive measure of AI model performance. Customizing a model specifically for this benchmark amplifies these concerns.
- Misleading Developers: The core issue is transparency. If the benchmarked version differs significantly from the standard Meta AI models available to developers, it becomes difficult to accurately predict real-world performance. This lack of clarity can hinder effective development and integration of the model.
The Problem with Tailored Benchmarks: Why Does it Matter?
Imagine purchasing a cryptocurrency mining rig based on advertised benchmark speeds, only to find the actual performance falls short in real-world mining scenarios. Similarly, in the AI world, misleading AI benchmarks can lead to wasted resources and misinformed decisions.
Here’s why tailoring models for benchmarks is problematic:
- Distorted Performance Snapshot: Benchmarks should ideally provide an unbiased overview of a model’s strengths and weaknesses across various tasks. Customization defeats this purpose, offering an inflated or skewed representation.
- Unpredictable Real-World Behavior: Developers rely on benchmarks to gauge how a model will perform in specific contexts. A benchmark-optimized version doesn’t accurately reflect the behavior of the ‘vanilla’ model, making predictions unreliable.
- Erosion of Trust: Transparency is paramount in the tech world, especially with rapidly advancing technologies like AI. Discrepancies between benchmarked and publicly available Meta AI models can erode trust in both the model and the company providing it.
Stark Differences Observed: Maverick on LM Arena vs. Publicly Downloadable Version
Researchers on X (formerly Twitter) have already highlighted noticeable differences between the LM Arena Maverick and the downloadable version. These observations further fuel concerns about the representativeness of the LM Arena benchmarks.
Examples of Discrepancies:
Feature | LM Arena Maverick | Publicly Downloadable Maverick |
---|---|---|
Emoji Usage | Excessive | Moderate/Normal |
Answer Length | Long-winded, verbose | More concise |
Overall Behavior | Potentially ‘cooked’ or over-optimized for conversational tasks | More balanced and general-purpose |
These seemingly superficial differences can indicate underlying adjustments made to the Maverick AI model specifically for the LM Arena evaluation. While conversational ability is important, optimizing solely for this aspect might come at the expense of other crucial performance metrics.
Okay Llama 4 is def a littled cooked lol, what is this yap city pic.twitter.com/y3GvhbVz65
— Nathan Lambert (@natolambert) April 6, 2025
for some reason, the Llama 4 model in Arena uses a lot more Emojis on together . ai, it seems better: pic.twitter.com/pf74ODXzTt
— Tech Dev Notes (@techdevnotes) April 6, 2025
Moving Forward: Transparency and Reliable AI Evaluation
The situation with Meta’s AI benchmarks and the Maverick model underscores the critical need for transparency and robust evaluation methods in the AI field. For developers, investors in AI-driven crypto projects, and the broader tech community, accurate and reliable benchmarks are essential for informed decision-making.
Key Takeaways:
- Demand Transparency: AI companies should be transparent about any modifications or optimizations made to models used for benchmarking.
- Critical Benchmark Evaluation: Users should critically assess benchmark results and consider the methodology and potential biases of different evaluation platforms like LM Arena.
- Focus on Real-World Performance: Ultimately, the true measure of an AI model’s value lies in its performance in real-world applications, not just benchmark scores.
As the AI landscape continues to evolve and intersect with cryptocurrency and blockchain technologies, staying informed about the nuances of AI model evaluation is paramount. The Maverick case serves as a potent reminder to look beyond headline rankings and delve deeper into the details behind the AI benchmarks we encounter.
To learn more about the latest AI market trends, explore our article on key developments shaping AI features.
0
0
Securely connect the portfolio you’re using to start.