Neurosymbolic AI Agents: Where Classical Meets Cutting-Edge
2
1
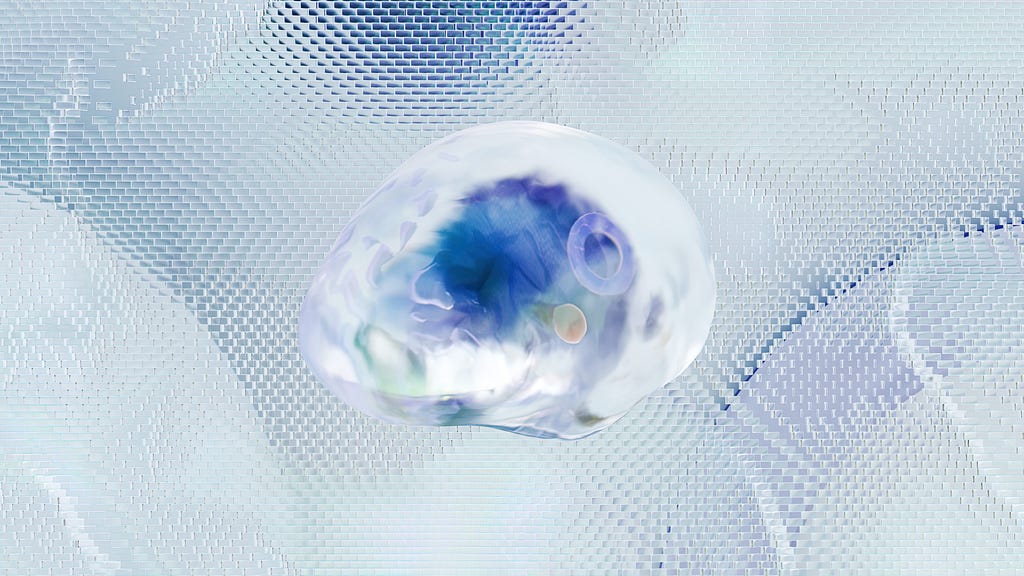
In the rapidly evolving realm of artificial intelligence, there’s a blend of old and new taking center stage: Neurosymbolic AI. For those on the cutting edge of AI innovation, this isn’t just another buzzword. It’s the nexus where classical AI meets modern neural networks, promising a leap in AI capabilities.
Understanding Neurosymbolic AI
To understand the significance of neurosymbolic AI, we need to go back in time. Classical AI, or symbolic AI, was all about predefined rules and symbols. This system used logic and structured knowledge representation to draw conclusions.
While it excelled in certain tasks, it was rigid and lacked adaptability.
Enter neural networks, the darlings of modern AI. These systems learn from vast amounts of data, allowing them to perform complex tasks like image recognition and language translation with astonishing accuracy. But here’s the catch: they often act as black boxes, with their decision-making processes being somewhat elusive.
Neurosymbolic AI seeks to combine the best of both worlds. It fuses symbolic reasoning’s structured, logical nature with the learning capabilities of neural networks. Combining the technology with AI agents can yield amazing results. Agents can learn, reason, and generalize from limited data, while also providing transparent decision-making processes.
Why Neurosymbolic AI Agents Matter
One major advantage of neurosymbolic AI is its ability to prevent AI systems from making false assumptions or seeing things that aren’t there. This is termed as ‘hallucinations’ in AI. Traditional AI can sometimes get things wrong by assuming patterns or trends that don’t exist. By mixing logic (symbolic reasoning) with learning (neural networks), neurosymbolic AI provides a clearer path. It uses logic to guide the AI, so it doesn’t jump to wrong conclusions.
However, there’s a balance to maintain. If we set too many rules, the AI might not learn well. So, it’s about ensuring the AI can learn but stays on the right track.
Neurosymbolic AI also has a wider industry impact:
- In industries like healthcare or finance, understanding AI decisions is crucial. Neurosymbolic AI offers that coveted transparency, making it easier to trust and implement AI solutions.
- While deep learning models require massive datasets, neurosymbolic approaches can make informed decisions with fewer data points by leveraging symbolic reasoning.
- Neurosymbolic AI agents can adapt to new scenarios without entirely retraining the model, thanks to their symbolic backbone.

Where can Neurosymbolic AI Agents make a significant impact?
- Healthcare: In diagnostic processes, having an AI model that can both learn from medical data and follow symbolic reasoning (for example: clinical guidelines) can be groundbreaking.
- Finance: Financial markets are data-rich but also operate on structured rules. Neurosymbolic AI can analyze data trends while also factoring in the regulations and standards governing the industry.
- Education: Educational software can be tailored to adjust to a student’s learning curve while also adhering to predefined curriculum guidelines.
Challenges on the Horizon
The road to perfecting neurosymbolic AI agents is not without hurdles:
- Integration Complexity: Merging two diverse AI approaches is no simple task. Researchers face challenges in seamlessly integrating symbolic reasoning with neural processing.
- Scalability: As with many AI solutions, ensuring that neurosymbolic models can scale without compromising performance is a concern.
- Interdisciplinary Expertise: Neurosymbolic AI requires expertise in both classical and modern AI, demanding a multidisciplinary approach that can be challenging to orchestrate.
The Future
Beyond the immediate commercial applications, there’s a bigger picture here. Neurosymbolic AI agents could redefine our relationship with technology. Imagine AI assistants that not only learn from our habits but can also engage in logical debates, or automated systems that not only detect anomalies but also provide structured, reasoned feedback.
As with any rising technology, collaboration will be key. Industry experts, researchers, and policymakers need to come together to set the path forward. Standardization, ethical considerations, and knowledge sharing will all play pivotal roles in shaping the neurosymbolic AI landscape.
Neurosymbolic AI Agents: Where Classical Meets Cutting-Edge was originally published in Fetch.ai on Medium, where people are continuing the conversation by highlighting and responding to this story.
2
1
Securely connect the portfolio you’re using to start.