History of AI: Part Six — The Revolution
1
0
History of AI: Part Six — The Revolution
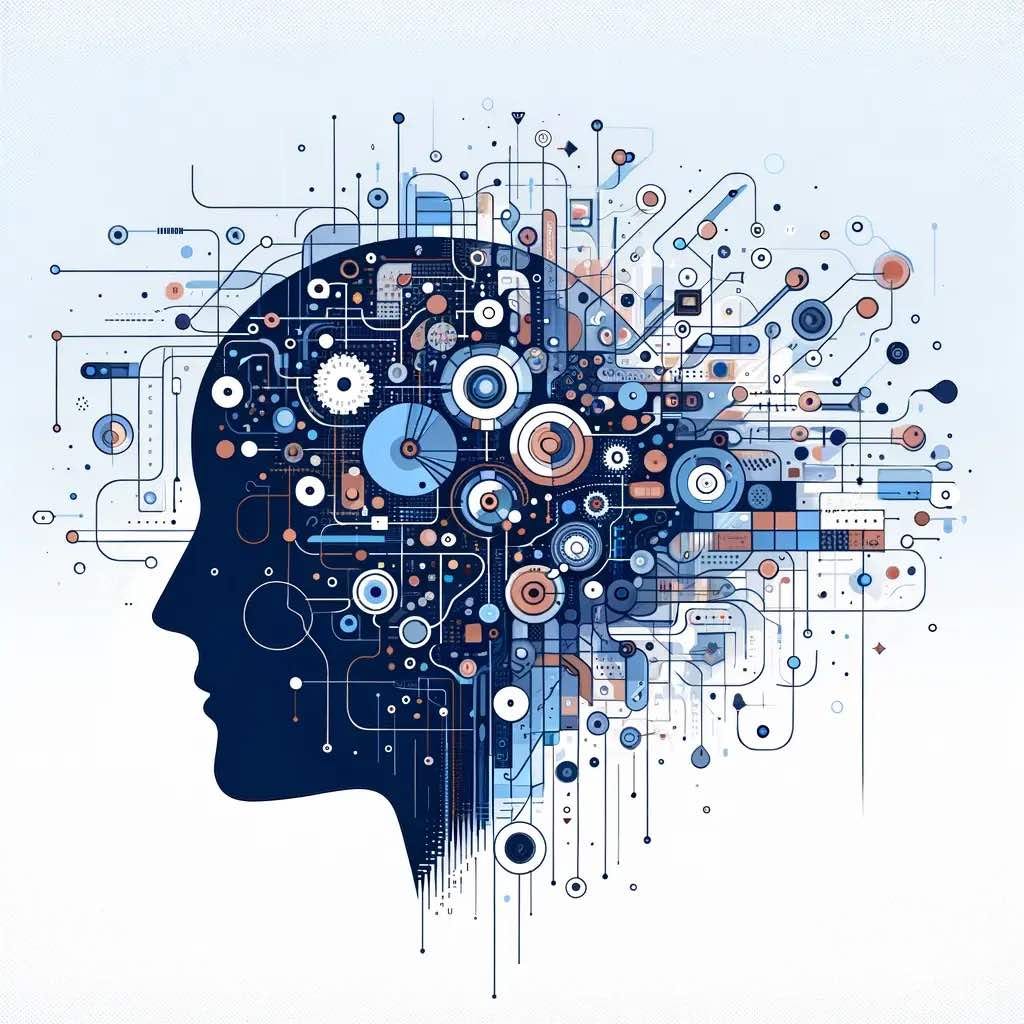
In this series chronicling AI history, we’re finally entering the 21st century.
So far, we have seen that AI embarked on a remarkable transformation over 50 years and slowly reshaped technology. Let’s now have a look at how it started reshaping our daily lives. Over the first two decades of this century, AI evolved from a mere concept to a reality.
The Emergence of New Applications
It was the early 2000s. The world survived Y2K — and was more excited about computers than ever. This is where new AI applications emerged. AI now was not only limited to research labs — but was slowly getting integrated into daily lives. It started helping with household gadgets to outer space exploration. For instance, in 2002, Roomba, a groundbreaking autonomous vacuum cleaner, was put to test in the markets. Just two years later, NASA’s Mars rovers, Spirit and Opportunity, made history by autonomously navigating the Martian terrain.
From simplifying daily chores to tackling the complexities of space exploration — AI was here. By the mid-2000s, AI was taking significant strides forward. One pivotal moment was in 2006 when the concept of “machine reading” was introduced. This breakthrough opened doors for AI systems to process and understand text independently, revolutionizing language comprehension.
This is where three key pieces of technology emerged: Big Data, Deep Learning and Large Language Models.
Big Data and Economic Impact
By 2009, around the time the world was rushing to recover from the great economic collapse — nearly every sector of the U.S. economy was managing colossal volumes of data. By all reports — the data averaged around 200 terabytes per sector. The decade saw a big change in how we deal with data. It became more available, computers got faster and cheaper. This allowed us to use advanced machine learning techniques. This period was all about the rise of big data, which transformed how industries handled information.
Instead of limiting ourselves to select samples, we began utilizing all available data for analysis. This comprehensive approach enhanced decision-making and optimization processes. Big data was distinguished by its large scale, rapid pace, diverse nature, intrinsic value, and accuracy. This necessitated the development of innovative processing models to fully capitalize on their potential.
Deep Learning: Advancements and Challenges
Deep learning emerged as an important piece of technology during this period. It modelled complex data abstractions using deep neural networks with multiple processing layers. Despite the Universal Approximation Theorem suggesting that deep networks weren’t necessary for approximating continuous functions, deep learning proved effective in addressing issues like overfitting, common in shallow networks. As a result, deep neural networks could generate far more intricate models than their shallow counterparts.
However, deep learning faced its own set of challenges. One significant issue was the vanishing gradient problem in recurrent neural networks, where gradients between layers diminished over time. Innovations such as Long Short-Term Memory (LSTM) units were developed to mitigate this problem.
State-of-the-art deep neural networks began rivalling human accuracy in fields like computer vision, as seen in tasks involving the MNIST database and traffic sign recognition. Furthermore, language processing engines, exemplified by IBM’s Watson, outperformed humans in general trivia, and advancements in deep learning achieved remarkable feats in games like Go and Doom.
Large language Models
In 2017, Google researchers wrote a paper titled Attention Is All You Need. This paper introduced the transformer architecture that improved upon the existing Seq2seq technology. The transformer architecture relied heavily on the attention mechanism, which had been developed by Bahdanau and others in 2014. This innovation laid the foundation for many subsequent advancements in AI language models. Large language models slowly started revolutionizing the field of artificial intelligence. In 2018, BERT, an encoder-only model, became widespread.
Then came GPT.
The GPT model was actually introduced in 2018 and met with lukewarm reception. It was GPT-2 in 2019 that garnered widespread attention. It was so powerful that OpenAI initially hesitated to release it to the public due to concerns about its potential for misuse. The model’s ability to generate contextually relevant text raised ethical questions about the responsible use of AI.
But then, right at the onset of the next decade — came GPT-3.
History of AI: Part Six — The Revolution was originally published in Fetch.ai on Medium, where people are continuing the conversation by highlighting and responding to this story.
1
0